Introduction
In today's world of data-driven decision-making, privacy has become a growing concern for individuals and organizations alike. As data analytics becomes increasingly prevalent, there is a mounting need to protect users' personal information without impeding innovation. Privacy by Design (PbD) is an approach that addresses this challenge by embedding privacy protection measures into the design of data analytics processes from the outset. In this blog, we will discuss the importance of Privacy by Design in data analytics and provide best practices for implementing it in your organization.
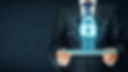
The Importance of Privacy by Design
Privacy by Design serves as a crucial aspect of data analytics because it:
a) Protects User Privacy: PbD ensures that data analytics processes adhere to privacy regulations and protect users' personal information, reducing the risk of data breaches and misuse.
b) Enhances Trust: Implementing PbD fosters trust among users and customers by demonstrating that an organization values and respects their privacy.
c) Ensures Compliance: By incorporating PbD principles, organizations can better meet the requirements of privacy regulations such as the General Data Protection Regulation (GDPR) and the California Consumer Privacy Act (CCPA).
d) Encourages Innovation: PbD promotes the development of privacy-enhancing technologies and innovative data analytics techniques that respect user privacy without compromising functionality.
Best Practices for Implementing Privacy by Design
To incorporate Privacy by Design into your data analytics processes, consider the following best practices:
a) Embed Privacy Early: Ensure that privacy is considered from the initial stages of a project, not as an afterthought. By integrating privacy measures into the design of data analytics processes, you can minimize the risk of privacy violations and costly fixes later on.
b) Minimize Data Collection: Collect only the data that is necessary for your analytics objectives, and avoid collecting excessive personal information. This reduces the risk of data breaches and ensures compliance with privacy regulations.
c) Implement Data Anonymization and Pseudonymization Techniques: Anonymize or pseudonymize personal data whenever possible to protect user privacy. This can include techniques such as masking, aggregation, and tokenization to ensure that data remains useful for analysis while minimizing the risk of identification.
d) Opt for Privacy-Enhancing Technologies: Use privacy-preserving technologies such as differential privacy and secure multi-party computation (SMPC) to conduct data analytics while maintaining user privacy.
e) Ensure Transparency and Accountability: Be transparent with users and stakeholders about your data analytics practices, including how personal information is collected, processed, and stored. Maintain accountability by implementing data protection policies and appointing a Data Protection Officer (DPO) to oversee privacy-related matters.
f) Regularly Assess and Update Privacy Measures: Continuously evaluate your privacy measures to ensure they remain effective in light of evolving technologies and regulations. Update your privacy policies and practices as needed to maintain compliance and protect user privacy.
Conclusion
As data analytics continues to play an increasingly important role in decision-making, Privacy by Design serves as a crucial safeguard for user privacy. By embedding privacy measures into the design of data analytics processes from the outset, organizations can protect users' personal information, comply with privacy regulations, and foster trust among customers. By following the best practices outlined in this blog, your organization can implement Privacy by Design effectively and responsibly harness the power of data analytics.